Having covered the very basics of machine learning (ML) in a previous post, I want to introduce you to an essential conceptual framework embodied in a simple graph called the “learning curve” or “training curve.” This graph can be made to aid evaluation of any ML project, and is invaluable for non-expert evaluation of a project. The learning curve is closely related to cross-validation, which we will also discuss.
Continue reading
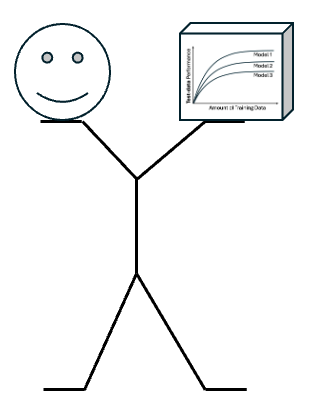